Where AI Gets Real: Multimodal Breakthroughs
How multimodal AI is sparking innovation in industries ranging from medical research, to air travel, to insurance
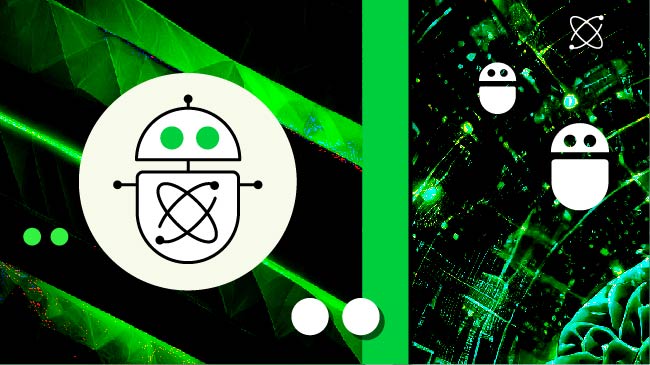
In December of 2022, OpenAI launched ChatGPT, enabling anyone from home cooks to CEOs to access AI and see the possibilities of the technology. As of April 2025, ChatGPT has grown to over 800 million active users, and OpenAI is on track to generate $11 billion in revenue by the end of the year.
AI is not new, but this excitement—enabled by the compute power provided by innovative firms including NVIDIA and AMD—enables capabilities and, more importantly, opportunities for AI to not only live up to the promises of the past but exceed our expectations.
Early versions of ChatGPT excelled at processing prompts and text, and at generating responses that were not just searching for answers but leveraging its vast training to respond and generate responses.
Want help reviewing an article about Multimodal AI? ChatGPT is great for reviewing, recommending updates, providing insight and providing style recommendations. Looking for help structuring a report, project plan or business proposal? ChatGPT and its competitors have become great assistants.
However, the world is not all about text. We mere mortals don’t think in text alone. We leverage multimodal input from a wide range of sources, including images, live activities, speech and other inputs that help contribute to our understanding and thoughts about the world around us.
The Promise of Multimodal AI
As Jensen Huang, CEO of NVIDIA, shared during his keynote at the GTC 2025 AI conference: “AI understands the context, understands what we’re asking. Understands the meaning of our request. It now generates answers. Fundamentally changed how computing is done.”
For AI to truly understand context the way people do, it must move beyond a single mode of input.
Imagine if you wanted to develop an AI-powered system for automated driving, yet all of the input you could provide was text-based. It wouldn’t work very well. Not having examples of navigating a car, visuals of traffic signs and sounds such as horns honking would make it challenging to develop an AI solution that could safely drive a car around the block or across the country.
AI providers including OpenAI, Meta, Google, IBM and others are delivering multimodal AI, and other AI solution providers delivering solutions whose models are trained on different types of input but generate responses beyond text. (See below a table of some of the leading multimodal AI solutions.)
It’s important to note that multimodal AI—blending multiple types of input—is different from multimodel AI, where organizations combine different specialized models to solve broader problems. (See below, “A Claification: Multimodal vs. Multimodel AI,” for a quick overview.)
McKinsey highlighted recent advances in a report issued in January. Google Gemini Live, the report said, “has improved audio quality and latency and can now deliver a human-like conversation with emotional nuance and expressiveness, Also, demonstrations of Sora by OpenAI show its ability to translate text to video.”
Real-World Breakthroughs
Multimodal AI has real-world implications to improve business and society. Not only do we improve responses from the larger language and foundation models by training them on these different sources of information, but the ability to train and generate multimodal responses can create real breakthroughs and innovations.
A recent Nvidia blog highlighted the work of BrainStorm Therapeutics, a health tech company that is using multimodal AI to “integrate data from cell sequencing, cell imaging, EEG scans and more” to accelerate the development of cures for diseases ranging from Alzheimer’s and Parkinson’s to hundreds of rare, lesser-known conditions.
Here are a couple other examples of how companies are finding innovation with multimodal AI:
- Qatar Airways has introduced an AI-powered virtual flight attendant that combines speech recognition, visual interaction and text-based responses to assist passengers by providing personalized, multimodal assistance for travel-related queries.
- A Fortune 100 insurer is boosting digital adoption with a multimodal virtual assistant leveraging a combination of voice, text and visual interfaces to increase self-service adoption rates and enhance customer satisfaction with intuitive, multimodal support options.
Challenges to Consider
As with any advancement in technology and AI, we need to consider additional challenges that multimodal AI can pose for organizations:
- For all types of input, consider the ownership of the content and their rights to control, publish and receive compensation.
- Consider the governance and privacy implications of shared content with customers, partners and suppliers. They deserve the same level of protection and trust as with other stored content.
- Multimodal AI brings about new cybersecurity threats. More types of input expand potential cyber issues and attack vectors. Hidden threats can be embedded across different types of data, making attacks more challenging to detect, causing organizations to rethink security practices to protect sensitive information and ensure AI-driven decisions stay reliable and trustworthy.
For organizations building their AI strategies, aligning multimodal capabilities with clear business priorities, as outlined in previous articles in our ‘Business of AI’ series, will be key to delivering sustainable value.
A Clarification: Multimodal vs. Multimodel AI
It can be confusing: multimodal vs multimodel AI.
- Multimodal AI handles different types of input—text, video, audio, and more—whether for prompting, training, fine-tuning or responses.
- Multimodel AI means using different specialized models for a solution. For example, an organization might combine OpenAI GPT for communication and a smaller fine-tuned model for product-specific knowledge.
- Example: In healthcare, specialized LLMs diagnose conditions, but a separate model translates diagnoses into patient-friendly language. Some solutions even integrate imaging models, making the final system both multimodal and multimodel.
Exploring Leading Multimodal AI Solutions
Below is a quick list of key multimodal platforms, including industry-specific solutions:
Provider | Focus / Industry Strength |
Google Gemini 1.5 | General-purpose multimodal AI (text, images, audio) for enterprise use cases |
OpenAI GPT-4 Turbo + Vision (and Sora) | Multimodal text-to-vision and text-to-video (creative industries, support, education) |
Meta Llama 3 +ImageBind | Open-source multimodal options for innovation and R&D (text, image, audio, sensors) |
IBM Granite Foundation Models | Enterprise-grade multimodal models (healthcare, finance, government focus) |
NVIDIA BioNeMo / Clara | Healthcare, pharma, life sciences—combining imaging, genomics and medical data |
Hugging Face Multimodal Models | Open-source models for healthcare, legal, finance industries |
Uniphore X-Platform | Multimodal customer experience (CX) solutions: voice, visual IVR and AI |
Viz.ai Clinical Solutions | Healthcare-specific multimodal diagnostics (stroke, aortic detection, etc.) |