IBM PowerAI Brings Together AI Solutions for Enterprises
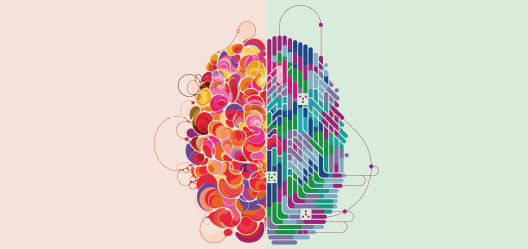
When you’re big, it’s tough to be quick. The artificial intelligence (AI) boom has sent some startups soaring into the future, but it’s left some big enterprises planted in the past.
“Every industry is being disrupted by AI startups—FinTech tools in the banking industry, self-driven cars in the auto industry—these new companies are using AI to disrupt the older companies,” says Sumit Gupta, vice president for IBM High Performance Computing, AI and Machine Learning.
“It’s ‘the art of the possible.’ All of these companies are proving what’s possible and forcing enterprises to look at emerging technologies to figure out how they can provide value to clients, improve services and optimize business processes,” Gupta says.
In the next year, enterprises will try to refine strategies and make bigger investments in AI—but many struggle with where to start. Enterprise teams need an AI platform that’s ready to work.
A Foundational Approach to AI
“I was describing a describing a high-end hardware system designed for AI to a major financial client in London,” Gupta recalls. “And the client said, ‘I like that, but can you guys give us the AI software? Can you guys precompile it? We don’t want to download and compile the open source.’ ”
The client explained that corporate policies didn’t allow the team to download open-source software. And every time the team downloaded approved source code, they spent up to a week compiling it and setting it up. Plus, they had to fend for themselves with no real support.
Gupta realized that his hardware team needed to expand its scope. “I remember coming back and telling the team, ‘Let’s make a software offering,’ ” he says. “We completely changed the plan.” And that’s how IBM PowerAI was born.
IBM Power Systems* hardware is the foundation for AI solutions based on open-source software. But enterprises often find that their environments are too complex and regulated to effectively use open-source platforms. Maintaining these platforms within enterprises can be time-consuming, and some open-source software licenses restrict commercial use or require that any intellectual property must become public domain.
As a result, enterprises gravitate toward commercial software. Gupta saw a clients’ need for a pre-configured platform for AI projects on an enterprise scale.
“We came along and presented an easy way to get started: ‘Here is a system with software that just works. You don’t have to go figure out what to buy, and you don’t have to download the software. Just install this and your data scientists are good to go,’ ” Gupta says.
But the PowerAI team went even farther. PowerAI augments the open-source platform with ease-of-use tools and performance libraries that help data scientists at enterprises to be more productive. “When we launched the offering for the first time, the groundswell of client interest was huge,” Gupta says. “That’s when we decided we were onto something.”
As enterprises move to embrace AI, PowerAI provides a comprehensive platform to get started with the AI solution that is leading the revolution in AI: deep learning.
Going Deeper than Machine Learning
Deep learning is a type of machine learning that holds powerful potential for organizations dealing with massive amounts of data, and IBM PowerAI is the first enterprise distribution of open-source deep learning software. “Enterprises should look at deep learning when they have enough data—as the amount of data increases, deep learning becomes more effective and accurate than traditional machine learning methods,” Gupta advises.
Traditional machine learning uses algorithms that are programmed to learn and adapt based on a particular domain and data. Deep learning is a raw neural network that’s almost domain-independent. Deep learning adapts to a domain by using models that identify important features in the domain’s data automatically, making it easier than traditional machine learning methods for data scientists to use.
“In many ways, it learns like we do. The problem is that we have decades to learn, whereas computer models are expected to learn within months,” Gupta points out. So, the PowerAI team developed enhancements to accelerate deep learning performance. “Deep learning software sometimes takes days and weeks to run, but we’re able to bring that down to hours. That enables data scientists to run many more learning iterations and significantly improves developer productivity.”
Deep learning is opening new doors for companies like RowAnalytics, a U.K.-based firm that specializes in Internet of Things (IoT) and healthcare solutions. RowAnalytics solutions sometimes need to process massive data that’s too large for traditional computing architectures. But PowerAI in conjunction with IBM Power Systems provides exclusive large memory support with deep learning that lets RowAnalytics analyze such data sets without breaking them up across multiple systems. The outcome is faster and more precise analysis.
The PowerAI team is also working with other companies developing machine learning and analytics software to add new capabilities. For instance, the team is working with Anaconda to tightly integrate tools and libraries popular among Python programmers. They’re also working with H2O to improve integration of the company’s machine learning libraries.
Getting Smarter
Getting started with AI and deep learning can be daunting for many enterprises. “People in industry have doubts of ‘I’m not sure this is useful for me. I don’t know if it applies to the problem I’m trying to solve. I don’t have the right talent to do the work.’ ” Gupta says.
Although the work takes time, the PowerAI team is building resources that help enterprises get up to speed as quickly as possible, capturing use cases and even reference architectures to help accelerate deployment. “Essentially, we’re becoming a repository for our clients to be able to learn, understand and get started with AI quickly.”
Today, deep learning solutions are already analyzing medical data to help improve patient treatment, analyzing internet data to thwart cyberattacks and analyzing financial data to better predict loan risks and detect fraud. “If we can improve fraud detection for credit cards—even by 1 percent—we’re talking hundreds of millions of dollars saved a year,” Gupta says.
“Enterprises have seen the potential—and now they’re getting the tools to explore ‘the art of the possible,’ finding ways to turn new technology into competitive solutions.”