PowerAI Vision Democratizes the Development of Visual AI Models
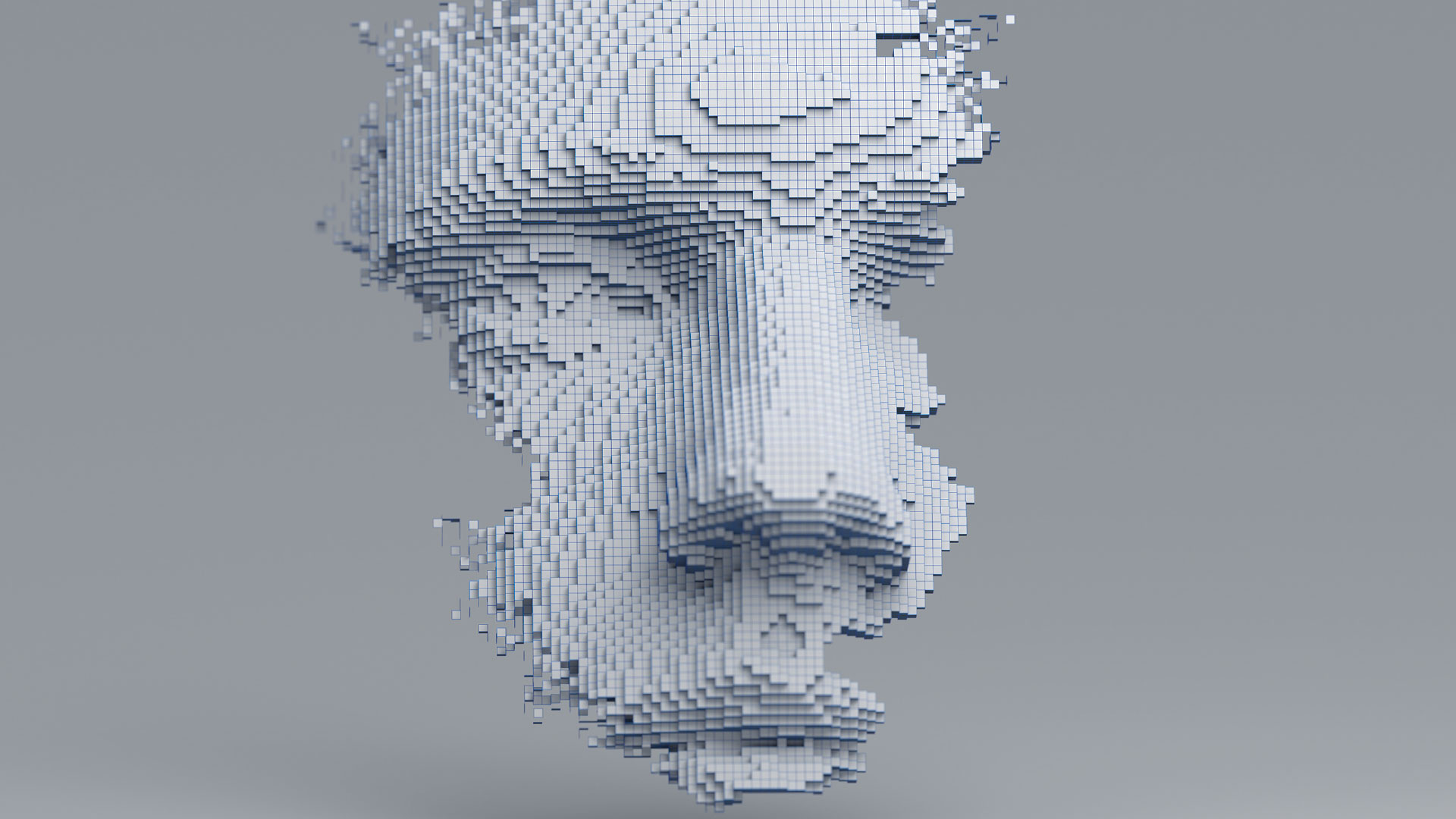
It used to be that only programmers actually programmed—that was their area of expertise. End users simply worked within the parameters set before them.
But then something happened that began to alter that relationship. Tools like spreadsheets allowed average employees to essentially do their own programming, building smart columns, rows and cells that could predictably crunch numbers for themselves and their co-workers.
A similar transition is now taking place in the AI world. Whereas data scientists were once the sole curators of AI models, tools like PowerAI Vision are handing some of that power down to everyday subject matter experts, no advanced degrees in AI required.
“You put a spreadsheet in the hands of somebody who’s not a programmer and they’re able to get value out of it,” says Michael Hollinger, chief architect for Machine Vision and Master Inventor, IBM Cognitive Systems. “We see the same thing happening with machine and deep learning. Our goal is to put that power into the hands of subject matter experts and let them use PowerAI Vision to solve problems on their own.”
Streamlining Model Training
This is in sharp contrast to how visual AI models were originally created. Previously, it may have required a full-time employee to sort through thousands of images to determine their validity compared to the problem that needed to be solved. This hands-on method, however, was time consuming and prone to inadvertent errors.
Designed to mitigate these experiences, PowerAI Vision streamlines much of this visual recognition process. For example, a person might only have to label 10 or 100 images and then have the system automatically label another 1,000 based on those previous entries. A quick look at the model then allows users to reject visuals that don’t fit the model’s projected goals. This approach—which may take only hours instead of days or weeks to complete—results in much more accurate models.
Hollinger built a demo to demonstrate this. He labeled five or six minutes of a high definition video clip that included 100 or so frames and then had PowerAI Vision automatically label another 500-600 frames. He then modified 50 to 100 of them, trained the system again and ended up with an accurately working model. He did this over the course of a single afternoon. “It’s very empowering to be able to do this,” he says.
Hollinger’s group also went so far as to rent space from a convenience store and hire actors to create a data set, which it then used to create a variety of models useful for retail settings, like detecting backups at a point-of-sale kiosk. If a large crowd begins to develop, the system will alert a manager that more assistance is needed behind the counter.
Potential Pitfalls
Caution does have to be applied when building these models, however. Hollinger cites a case where a Ph.D. candidate was defending her dissertation by having an AI-enabled drone fly around her school’s campus and perform certain tasks. Initial testing went well in the spring of that year, but when she had to actually defend her dissertation in the fall, the drone promptly flew into a tree.
The reason, according to Hollinger: “It had initially learned that a tree had to have leaves, but in the fall—in December, in this case—there weren’t any leaves on the tree. It hadn’t been trained to recognize that difference. Happily, she did end up getting her Ph.D.”
Always Adapting
This anecdote actually demonstrates how the power of PowerAI Vision truly comes into play. It allows users to quickly iterate developed models, improving them so users can easily react to changes in the field. As a result, a model can be rapidly updated to accommodate, for example, summer-to-winter alterations in retail-employee uniforms—from short sleeve to long sleeve—to ensure the retailer can continue to differentiate between customers and employees no matter the season.
PowerAI Vision also has the capability to detect video action as it happens, such as whether someone is picking up or putting down an item. As Hollinger explains, “We can label actions such as removing an item from a customer’s cart, scanning or weighing the item, and putting it in the bag. So, there should be those three steps, but what happens if someone picks up an item and puts in the bag without scanning or weighing it? Was it a mistake or was it theft? We can now use video action detection to provide an alert that something odd happened here.”
The potential uses for PowerAI Vision are seemingly endless. For example, the Hong Kong International Airport is using the tool to help the airport manage its new terminal expansion, with use cases such as finding out-of-place baggage carts and improving worker safety.
Just recently, support for a medical file format related to imaging was added to PowerAI Vision. Radiologists can now use that capability to build models based on images and discover questionable or problematic cases, thereby spending less time examining normal scans. Significantly, these AI models are merely making suggestions, with the medical practitioners being the final arbiters as to where they should focus their efforts and whether they should pay extra attention to certain cases.
Democratizing AI
Thanks to tools such as PowerAI Vision, the process by which AI models are developed is becoming democratized, no longer the sole purview of data scientists. Indeed, model development is also becoming increasingly collaborative, with subject matter experts laying the foundations of models and perhaps turning to data scientists if something seems amiss.
“PowerAI Vision is enabling analysts with primary expertise to work with and label data sets, label video or label images and enable them to actually train a model and have that model validate that it itself works,” Hollinger says. “If needed, they can then work with data scientists, who enter the advanced mode to take a deeper dive into what might be causing an issue. We want to make that interaction as easy and seamless as possible.”