The Business of AI: A Look at the Different Types of AI and Their Value
In the second article of his series, "The Business of AI," sales and marketing leader Brian Silverman further explains the various kinds of AI and their use cases
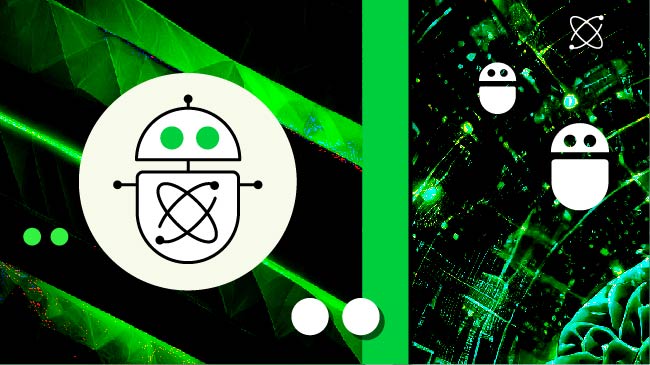
This is the second article of the series, “The Business of AI.” Here are the rest of the articles that have been published so far:
1. Focus on ‘The Business of AI’ to Move From Hype to ROI
3. From Foundation Models to Large Language Models
4. Current Applications and Data Fuel AI Innovation and Solutions
The “Business of AI” series began by emphasizing the need to move beyond AI hype and focus on achieving real business value and return on investment (ROI). In this article, we will focus on how to uncover the ROI potential of AI investments by choosing the right type of AI for an organization’s requirements and use cases.
The first step is to recognize that not all business use cases are best solved by leveraging AI. It is important to match the best solution for an organization’s business requirements, including current investment in capable systems, applications and data. For example, tasks such as automating payroll processing or inventory management are often better handled by existing software systems rather than the latest AI solutions. In addition, these systems of record often provide the important data necessary to fuel future innovative AI use cases.
Let’s review the different types of AI discussed in the previous article in more detail, including their value, potential returns and when to consider each type for your AI business use cases.
Generative AI
Generative AI (GenAI) is called “generative” because it can generate new content and create responses to support AI requirements and opportunities. GenAI leverages advances in AI including deep learning and transformative training of foundation models and large language models (LLMs). LLMs are trained on broad information and, in contrast to traditional AI, are built to support a variety of use cases for current and future business AI opportunities. (We will discuss LLMs and foundation models in more detail in our next article.)
One form of GenAI, AI assistants—such as OpenAI’s ChatGPT, Meta’s AI assistant, Microsoft’s Copilot, Anthropic’s Claude and Google’s Gemini—leverage their organization’s publicly available foundation models. They can assist employees by improving their efficiency and effectiveness across many tasks, from editing emails to developing code, and much more, providing greater “return on employee.” These assistants deliver value to improve employee productivity and happiness, but it can be difficult to calculate the actual financial ROI.
Leveraging GenAI tailored for business opportunities and return for an organization requires a different approach.
For many AI use cases, the most efficient and effective approach will be to leverage the growing number of available foundation models and LLMs, including those available from the providers of the assistants mentioned above. Developers can access the foundation model provider’s APIs, incorporating prompts into their applications. Though publicly accessible, many of these models can be deployed privately so that they can be securely trained and fine-tuned with proprietary and confidential data that will support unique AI applications, opportunities and use cases.
The other option is to build your own GenAI solutions, including developing and training your own LLMs. Capital One, for example, is eagerly leveraging AI, and has developed a proprietary GenAI tool that helps customer service agents access information to resolve customer questions more quickly and efficiently.
Smaller organizations may face challenges such as limited access to skilled personnel or high development costs preventing them from developing their own AI applications. However, there is much they can gain by leveraging publicly available models and assistants, by carefully planning, choosing the right use cases and leveraging available AI technologies.
There are new GenAI solutions focused on particular business requirements coming online every day. For example, Uptake, an AI-powered solution for fleet maintenance, leverages data and sensor feeds from a number of manufacturers to help transportation companies and others provide ideal maintenance to their fleets. (See the the AI Solution Directories addendum below.)
To prevent their proprietary information and confidential data from being accessed by others, organizations of all sizes need to be sure they are not sending it to publicly accessible and trained LLMs, foundation models and applications.
Traditional AI
Traditional AI focuses on machine learning and expert rules-based systems to leverage data, machine learning and training, enabling traditional AI systems to deliver accurate results consistently for specific requirements.
For example, consider a manufacturer looking to consistently analyze warranty claims to improve their support process and product quality. The system is trained on internal data such as customer support information. The data and AI engineer, along with business professionals, train the system to detect and analyze issues with warranty claims. This accelerates recommendations for improving the warranty process and identifying quality-control issues, thus reducing claims and improving customer satisfaction.
When evaluating your requirements for traditional AI compared to GenAI, consider that traditional AI can deliver higher accuracy for specific use cases and requirements, while GenAI can hallucinate and be less consistent with answers. If your use case primarily leverages the data you have in-house for a specific business process or requirement, traditional AI may be quicker to deliver true results than GenAI.
Don’t forget to balance the cost vs. benefits of creating and running your AI Applications. Traditional AI may be more costly than non-AI systems but, depending on specific requirements, can be less expensive than GenAI.
Agentic AI
Agentic AI is the next AI model to emerge from GenAI. AI agents are capable of completing complex work assignments autonomously and collaborating with other agents, causing some analysts to call them digital workers.
Solutions such as IBM’s watsonx.ai flows engine are coming online to create and orchestrate AI Agents that can independently navigate complex tasks, collaborate seamlessly and enhance workflow automation for optimal efficiency and decision-making.
Agentic AI-specific solutions such as TechSee, an autonomous customer service agent, are already available. TechSee’s Sophie is an agentic AI plugin that not only identifies problems but also interprets context and takes action to solve them.
While adoption is still in its early stages, businesses are increasingly exploring agentic AI to address autonomy demands in dynamic environments, promising broader implementation in the near future
So, when developing an AI strategy and considering which AI technology to leverage for a particular use case, organizations should consider these key points:
- Not all business opportunities, computing opportunities or use cases require AI. Leverage the best solution to match business requirements, including current capable systems, applications, data and investments.
- To provide the best return on their AI investments, organizations need to move beyond the hype of GenAI to choose the right type of AI for their particular requirements.
- GenAI solutions are great at taking the data of your systems, as well as unstructured information, and leveraging LLMs and foundation models’ broad training to provide unique solutions for current and future AI use cases.
- Traditional AI is excellent when you have a use case requiring accuracy, allowing you to leverage your own data to train an AI model.
- Agentic AI is the next phase of AI, built on the GenAI and deep learning planning to take AI to the next level, moving from assisting human work to AI agents capable of being true digital workers completing complex assignments.
- It is important to recognize that you may leverage different types of AI and deployment choices for each opportunity or use case.
- AI, in any form, is not inexpensive, and it is essential to conduct a thorough cost-benefit analysis to understand the options and select what is best for each business use case. Measuring ROI accurately ensures that AI investments align with strategic business objectives.
The next article in the TechChannel “Business of AI” series will explore LLMs and foundation models, including how to identify and select the right model and approach for your GenAI strategy.
AI Solution Directories
Every day there are new AI solutions coming to market that address specific industry and cross-industry tasks. They provide advanced AI capabilities, and for many organizations they can provide the quickest return on value.
For example, a common one that most already know about is Grammarly, which provides spelling and grammar recommendations to improve content, from emails to this series of articles.
Organizations developing a comprehensive AI strategy should consider available AI solutions in addition to developing their own AI applications in-house. The following list of directories and guides can help you find them.
Simplilearn: 24 Artificial Intelligence Applications in 2025: This site lists a range of uses for AI, from e-commerce to entertainment.
Gartner Peer Insights: This platform provides reviews of various GenAI apps from users. You can filter by rating, industry and company size to find the right app for your needs.
NVIDIA’s Catalog of Generative AI Apps: This catalog focuses on enterprise applications of Generative AI, including those for cybersecurity, computer-aided engineering, and robotics.
Marketing AI Institute’s List of Generative AI Tools: This list includes a variety of tools for marketing and business, such as those for content creation, SEO, and social media ma
Massachusetts Library System’s Guide to Generative AI Tools: This guide provides a comprehensive list of Generative AI tools, including those for audio and video generation, as well as prompts and detectors.
Futurepedia: Extensive, searchable directory with good filtering options. Strong on GenAI.
There’s An AI For That: Excellent for task-based searches. Many generative AI tools, but filters for other types exist.
AITopTools: Wide range of AI tools with decent filtering. Not as deep on individual entries as some others.
Toolify.ai: Focuses on categorizing AI tools by use case. Search is okay, but browsing categories is a strength.
Considerations you may want to review as you do your own search:
- Are they hosted only, or can they be deployed in your own IT infrastructure? This could impact data privacy and confidentiality, as well as legal and industry compliance.
- What are their data policies, and will they use the data you utilize to further train their app? And will this data be available in the public domain?
- How many customer references are available, and how good is their fit with requirements?
- Is the solution accessing and leveraging publicly available foundation models and LLMs? And if so, how do they incorporate their data and confidentiality policies into their own security policies and commitments?
- How customizable is the solution? To what extent can it adapt to an organization’s unique requirements?
- How well does the solution scale and perform? Do they have a service level agreement (SLA) in place?
- How do they charge for the solution and how transparent are the costs? What causes costs to go up? What are their recommendations on planning?