IBM Machine Learning for z/OS Gives Clients the Tools to Make Better, Faster Decisions
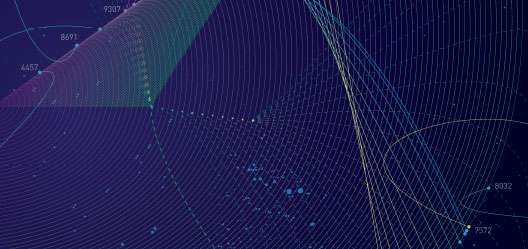
A customer seeks a home-improvement loan to accommodate her growing home-based business. The bank’s loan officer looks at her credit score. It’s not bad, but it also suggests there’s a high probability she won’t be able to pay back the loan. The loan officer says as much to his manager.
Not long thereafter, his boss returns the application with the word “approved.”
This leaves the loan officer scratching his head. In fairness, his manager had access to more information than just the customer’s credit score. With the help of the bank’s data analyst, he was able to examine data concerning the customer’s saving and investment patterns, income, accounts and even information about her business’s social media postings. And based on this data, as well as comparison to customers that fall in similar parameters, it turns out the customer is actually a good risk—and thus a good opportunity for the bank.
“Using machine learning, data scientists can uncover new insights from their data to support better outcomes. These innovative insights will generate new business opportunities.”
—Terrie Jacopi, IBM z Systems analytics offering manager
This is a simple example of an organization using internal and external data to derive better insight. Data and analytics have become more integrated with the way businesses operate. But analyzing data to make it useful remains a huge challenge. That’s chiefly because of the amount and sources of the data.
In February, IBM introduced Machine Learning for z/OS*. This new offering transforms the IBM z Systems* mainframe into a machine learning platform. IBM Machine Learning for z/OS allows clients and their data scientists to quickly create, deploy and continuously monitor a high volume of analytic models.
IBM Machine Learning’s capabilities are helping data scientists identify patterns in historical data, build behavioral models from those patterns, and make recommendations based on those behavioral models to help predict the most accurate outcomes. These models can help banks, governments, insurers, manufacturers, retailers and service providers make better decisions. And like the earlier example, they can use machine learning to better understand their clients and offer services or products that are highly targeted to individual needs. What’s more, IBM Machine Learning continuously monitors these models to ensure they remain accurate and useful.
Thinking Through the Data
First, a quick definition: Machine learning is a type of artificial intelligence that enables a computer to learn without being explicitly programmed, thus requiring less human intervention. It uses what it learns to anticipate or predict a behavior or outcome.
“When data scientists are solving a business problem, the data within their organization often holds the insights they need to find a solution,” says Terrie Jacopi, IBM z Systems analytics offering manager. “The broader the set of data they can evaluate, the better the outcomes. They have to understand all of the data sources that are relevant to solving the problem and then determine the best approach.”
Again, the big challenge is the amount of data out there. Insights need to be gleaned not only from an organization’s own information, but also from outside sources, such as social media and mobile devices. That external data can originate from current or potential customers. For data scientists and their organizations, channeling that flood of data can be a massive headache. It’s also an opportunity. “If you can take data that’s core to your business, like transactional data that originates on IBM z Systems, and combine it with external data, the insights you get about your clients are phenomenal,” Jacopi says.
To develop and exploit those insights, data scientists build behavioral models based on patterns within data. “Traditionally, data scientists do a lot of manual, iterative model development.” Jacopi notes. “Numerous algorithms might be relevant, and they have to figure out which approach would be the best. Data scientists spend a great deal of their time identifying the best model by trial and error, which takes time away from addressing the business challenge.” And time works against successful model development. Models can degrade as business, markets and data change—which, as most organizations know, can happen rapidly.
These are the types of data analysis puzzles IBM Machine Learning has been designed to help solve.
Model Making—and Remaking
One of the capabilities offered by IBM Machine Learning for z/OS is the Cognitive Assistant for Data Scientists. This IBM-patented technology helps eliminate the trial-and-error process so data scientists can consistently and more easily choose the best model to address business problems.
A second key IBM Machine Learning capability is Hyper Parameter Optimization (HPO). “At times, a data scientist can have as many as 100 parameters to consider,” Jacopi says. “This makes it time-consuming and difficult to determine which are the most critical parameters to optimize the model and gain the best outcome.” HPO aids the data scientist in selecting the best parameters to optimize the predictive capabilities of the model.
Once the model has been tested to ascertain that it’s providing useful, accurate predictions, putting that model to work “is a push-button deployment,” Jacopi says. Clients have told her it can take anywhere from three to six months to deploy a model using traditional methods. With IBM Machine Learning for z/OS, models can now be deployed in seconds. If the model needs to be updated, that new iteration also can be instantly deployed. As a result, she adds, the data scientist can “address business problems faster and deliver greater value.”
In addition, IBM Machine Learning for z/OS incorporates and employs a continuous monitoring and feedback loop. As new data arises, a data scientist must determine whether the model developed months ago is still providing valid predictions. IBM Machine Learning for z/OS includes a dashboard that provides a real-time view of model accuracy. When deploying a model, a data scientist can set a threshold (e.g., if the model degrades below 90 percent). IBM Machine Learning for z/OS will notify the data scientist if and when the model accuracy falls below this level. He or she then can decide whether to refresh the model with the new data to improve accuracy.
IBM Machine Learning for z/OS also lets application developers use RESTful APIs to “call” a behavioral model to get a prediction. For example, if the business is struggling with fraudulent credit card transactions, a data scientist using IBM Machine Learning for z/OS can develop a credit card fraud detection model and deploy it. “RESTful APIs make it simpler for the application to leverage the output from the model,” says Jacopi.
New Insights, New Opportunities
Organizations can use machine learning-generated models to make operational improvements. One of Jacopi’s clients, a healthcare company, has been seeking ways to help patients improve their health. The client has a great deal of data on each of its customers, including lists of prescribed medications and whether these patients take their medications regularly. Based on this data and other information, the company can use machine learning to better understand—and thus improve—each customer’s distinctive health behaviors.
“It’s looking for better ways to pull together large volumes of complex data, make better recommendations and improve client health,” Jacopi explains. “They want to help their customers make better choices and positively influence their own healthcare. In this scenario, patients lead healthier lives and costs are lower, so everyone wins.”
Yes, digital data can be overwhelming. But data is also highly valuable, and it’s crucial to an organization’s success in the digital age. Big data not only allows enterprises of all kinds to better understand their customers—it can also help them better engage with and serve them.
“With IBM Machine Learning for z/OS, data scientists will be even more productive and valuable to the organization,” Jacopi says. “Using machine learning, data scientists can uncover new insights from their data to support better outcomes. These innovative insights will generate new business opportunities.”