Accelerating Clinical Trials With Big Data Analytics
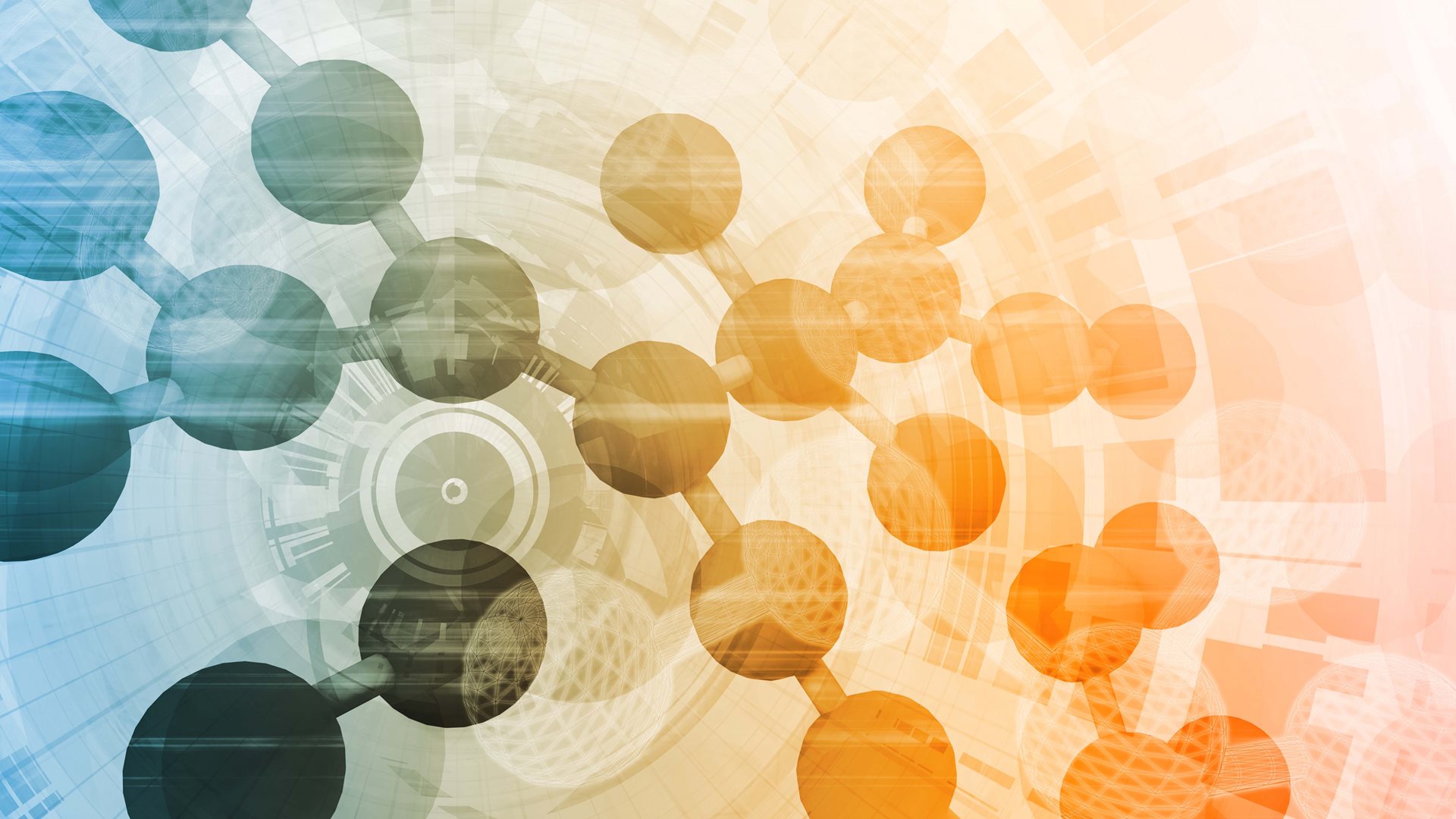
We’ve seen several groundbreaking scientific discoveries and technological breakthroughs over the last few years—from the second coming of mRNA, to gene therapies and cell therapies, and of course—emerging COVID-19 treatments and vaccines. If there’s anything this pandemic has taught us, it’s the importance of science and medical research.
But these groundbreaking discoveries didn’t emerge out of thin air, they are the result of many collective years of hard work by researchers both in the lab and clinic. According to Raj Indupuri, CEO, eClinical Solutions, a significant difference in the development of the COVID-19 vaccine versus other medicines that take 15+ years to complete was collaboration among academic researchers, government organizations and the life sciences industry. “We’ve seen more collaboration among different stakeholders in the healthcare industry,” he notes. “Companies like Moderna and Pfizer worked collaboratively with governments, regulators and research institutes around the globe in order to bring the vaccine to the clinic in record time.” Indupuri also notes that vaccine developers, aided by government support and collaboration were willing to take risks and adopt technologies at a much faster pace for these programs.
Over the last two years, reflects Indupuri, companies in the life-sciences industry have been investing more into digital transformation initiatives, with an emphasis on modernizing technology infrastructure. “That means more investments into data infrastructures, whether it’s to handle big data, deploy advanced analytics and machine learning models. It’s leading into larger investments into data ecosystems, along with modernizing analytic capabilities, which is much needed to tap into the data that’s already been collected and is available.”
However, one less fortunate trend in the space is: “The amount of data chaos is increasing significantly,” Indupuri notes. “So, we must find ways to tap into this data, transform this data into valuable insights.”
Data Management in the Healthcare Industry
This brings us to the importance of data management in the healthcare industry. Data management is a very broadly used term, but it takes on a particularly important meaning in our space: “Data management has a very critical and important function as it relates to clinical development or clinical trials—because you have all of these streams of data to be handled,” Indupuri says.
In the midst of the pandemic, there’s also been added pressure to bring research to patients. “In this case, we’re trying to collect data directly from patients, whether it’s from devices or applications. We have multiple streams of data and lot of external data sources such as labs, biomarkers and data from patients as assessments” says Indupuri.
With all of this incoming data, effective data management becomes increasingly important. Data must be monitored, curated and cleaned, to ensure it’s high-quality and reliable. “Data management plays a critical role in the value chain, and if you want to reduce downstream cycles times, you need high quality data,” Indupuri says.
Accelerating Clinical Trials With AI, Machine Learning and Big Data Strategies
That’s where big data, advanced analytics, AI and machine learning come in. These strategies can help reduce overall clinical trial cycle times needed to bring emerging research to fruition. “With COVID-19, and everything else going on, I think now everyone understands the importance of accelerating clinical trials leveraging technology” notes Indupuri.
Clinical trials are becoming increasingly complex, and the amount of data collected and used to conduct the trials only continues to increase. In addition to this growing data for new research, there is already a plethora of data from past clinical trials that can be harnessed. Big data, advanced analytics, AI and machine learning can help tap into this data, transforming it into valuable insights—accelerating research, and bringing medicine to patients faster.
For example, eClinical Solutions works with big pharma companies around the world, many of whom are leveraging data analytics to accelerate development of medicines. “We’re helping companies tame this data chaos,” Indupuri adds. “We provide a way for companies to take control of and transform this raw data into something meaningful, while eliminating some of the inefficiencies within clinical research and clinical development.”
Taking an Agile Approach
Like any corporate strategy, implementing advanced analytics, AI and machine learning techniques should be thought through and planned out. “Taming this data problem has been always complex and challenging,” Indupuri says. “We always recommend taking an agile approach, to deliver quick wins without trying to boil the ocean.”
When organizations invest too heavily and aggressively in building out massive data lakes, it can become a point of failure. Instead, companies should take a modular approach. For example, you could build a data lake for clinical research data, and one for commercial R&D data. But, notes Indupuri, the key is to take a platform-centric approach to build an interoperable ecosystem where these data hubs or data lakes can talk to each other, rather than building one massive infrastructure to solve every use case.
This is why it’s important to identify specific use cases that can be solved quickly and deliver quick wins that offer faster time to value—tying directly to support process re-engineering, and workforce transformation. “It’s ultimately about people, process and technology,” says Indupuri.
For Indupuri, this trio is key in transforming data management in the space. “When we talk about data or advanced analytics or machine learning, I truly believe a competitive advantage will come from a combination of both human element and automation,” he says. “We have to tap into data managers’ domain knowledge, to train the machine learning model, so the model can improve. It’s not just automation—it’s the people, the process and the technology working together.”
Future Big Data Trends
The benefits of effective data management through advanced analytics is clear, but where do we go from here? Three to five years from now, Indupuri predicts that there will be a slow but sure, significant adoption of big data, AI, advanced analytics and machine learning. “In the future, it’s going to be pervasive across clinical development and overall in life sciences. If you’re talking about data management where we actually have experience, it’s going to be widely adopted as well.”
Whether its discovery, research, or identifying patients for clinical trials, advanced analytics will be there to accelerate cycle times and help solve the data problem—now, and into the future.