Layering the World
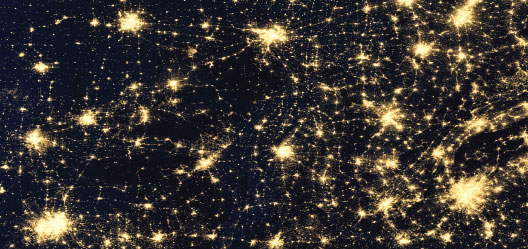
Microscopes have advanced so far since their inception, they may soon be able to see the small scale in almost infinite detail. But the large scale? It seems much easier to visualize—look up at the sky—but when big, complex data gets thrown into the mix, that may not be the case. The sky is blue, sure, but what impact does that have?
To help answer questions such as those, Hendrik Hamann, research manager for Physical Analytics, IBM Research, and colleagues are working on what they call a “macroscope.” This technology will allow users to employ, for example, satellite imagery of the earth, climate conditions, Internet of Things (IoT) data, population rates and water conditions to determine when and where to grow what types of crops.
“To conduct space and time analyses, you need access to all of these different layers of information at the same time and in the same space. That’s exactly what the macroscope is promising.”
—Hendrik Hamann, research manager for Physical Analytics, IBM Research
And this is only the beginning. By layering geospatial and assorted other data, big-picture questions will soon be answered on a level almost as detailed as what a microscope can expose, all easily searchable and discoverable.
IBM Systems Magazine (ISM): Information in the virtual world has already been pretty well indexed and made. How would a similar approach to the physical world work?
Hendrik Hamann (HH): We can search 45 billion webpages in less than 0.5 seconds because we’ve done a fantastic job of indexing available digital data, including data from social networks and relations. The macroscope is aiming to do this for data from the physical world, which is generally spatiotemporal data. It allows you to bring information together that’s in space and time. So you can pretty easily find everything on the web today, including locations that are close to where you live, for example.
But it’s a completely different task to ask where you’d like to live. You can see in a magazine the 10 best places in America to live, but that’s very nonsystematic. So you have to develop a profile: “I want to live right where there are a lot of Italian restaurants, where it’s not too hot, it’s not too cold but I would still like a little bit of the seasons.” Then you would search a large spatiotemporal data set. With the macroscope, we’ll make all of that data much more searchable and discoverable.
ISM: Stupid question, but what makes it so difficult to index space and time?
HH: Anything in the physical world happens in either space and/or time. That may sound like a trivial thing, but when you really start thinking about information in space and time, it’s not that easy. For example, with space, there are different map projections because the earth is neither round nor spherical, etc. And if you want to link things in space, things are moving. Continental plates are moving in space and moving in time, so actually making links in space and time is very complex.
Data size creates another issue. Global weather data, for example, represents tens of terabytes every day. That makes it very difficult to make information such as this searchable and discoverable. We have to make big progress towards digitizing the physical world through the IoT and then make that information much more accessible, discoverable, etc.
ISM: How would you collect the necessary data to make the macroscope feasible?
HH: We, for example, subscribe to pretty much every satellite service available. Satellites are producing images, some every 15 minutes, over large fractions of the globe. The U.S. government has a couple of very nice satellites that anyone can access, but the data is sitting in large files and is completely undiscoverable. So, for example, if I wanted to search for something in satellite images files, which are typically gigantic, I would have to open and look through each one of them. This is like in the old days when, if you went to the library, you had to read a whole book to find out if there was something you were searching for. That is where we are now with huge spatial data, which is why we want to make all this data indexable and discoverable. That’s where the power of the macroscope comes into play.
ISM: Is machine learning involved in this data discovery?
HH: One interesting aspect of this is the curation of the data. The truth about big data is that a lot of time is spent on data curation rather than analysis. Data curation includes indexing, cleaning the data up and understanding it. Machine-learning aspects are involved in all of this. But in this case, you want all of the layers of spatial and temporal information. One layer might be the weather, another population, maybe the spatial distribution of Twitter feeds, and another might include certain IoT sensors. All of these layers of information are linked in space and in time, and you index it in space and time. That’s really the essence of what you’re doing to make all of this information searchable, relatable, contextual, which is where a lot of interesting discoveries will come from. Some of the discoveries will include the use of complex machine-learning methods.
ISM: Do you have an example of how a company might use this approach to layer space and time data?
HH: Sure. We worked with Gallo Winery on irrigation analytics, calculating the optimal irrigation for their grapes to enhance water-use efficiency and grape quality. Of course, you need many layers of information to understand this. You need a layer about the soil—actually you will need many layers of soil because of its contents, like its pH, etc. You also need to know something about the weather, both present and future. You need to know about the wind, sun and then the water loss associated with that. You need to know something about the density of the canopy. That comes from satellite observations.
Typically, you’d have to do very complex calculations between these different layers of information. The beauty of the macroscope is all of these layers are linked, which allows you to conduct very efficient types of calculations and in the scale you need. In this case, Gallo ended up reducing water usage by 25 percent and the crop yield went up by 26 percent. And this was without a macroscope, but rather, the realization that we needed to fuse all of these layers of information together.
ISM: Would this apply to agriculture in general?
HH: Well, we know Earth’s population is increasing, but additional land on which to grow our food isn’t. We also know, most likely, we’ll have less water to use in crop cultivation. You combine these three issues and I think it becomes clear why it’s essential to use all this data for optimizing agriculture. So we need smarter data systems to help us with the decisions such as where to grow what, how to optimize crops, where the demands are, how commodities will be transported. Similar opportunities exist in any number of industries, including clean-energy generation and even astronomy, where you might be able to predict asteroid collisions based on layered data from different telescopes. The key to all of this is data, and geospatial data will play a huge role in that.
ISM: What’s the key takeaway from the work you’re doing?
HH: The key point of the macroscope is making this data accessible, discoverable and usable. As we’ve discussed, there’s a lot of spatial satellite data available. The U.S. government has a gigantic archive, but it hasn’t been used as a whole. We’re just looking at the tip of the iceberg in terms of the available information we can get from all of that data, with the primary reason being the complexity of the technology. The data hasn’t been curated. It hasn’t been indexed. To conduct space and time analyses, you need access to all of these different layers of information at the same time and in the same space. That’s exactly what the macroscope is promising.