The New IBM ESS 3500 Unlocks the Power of Data
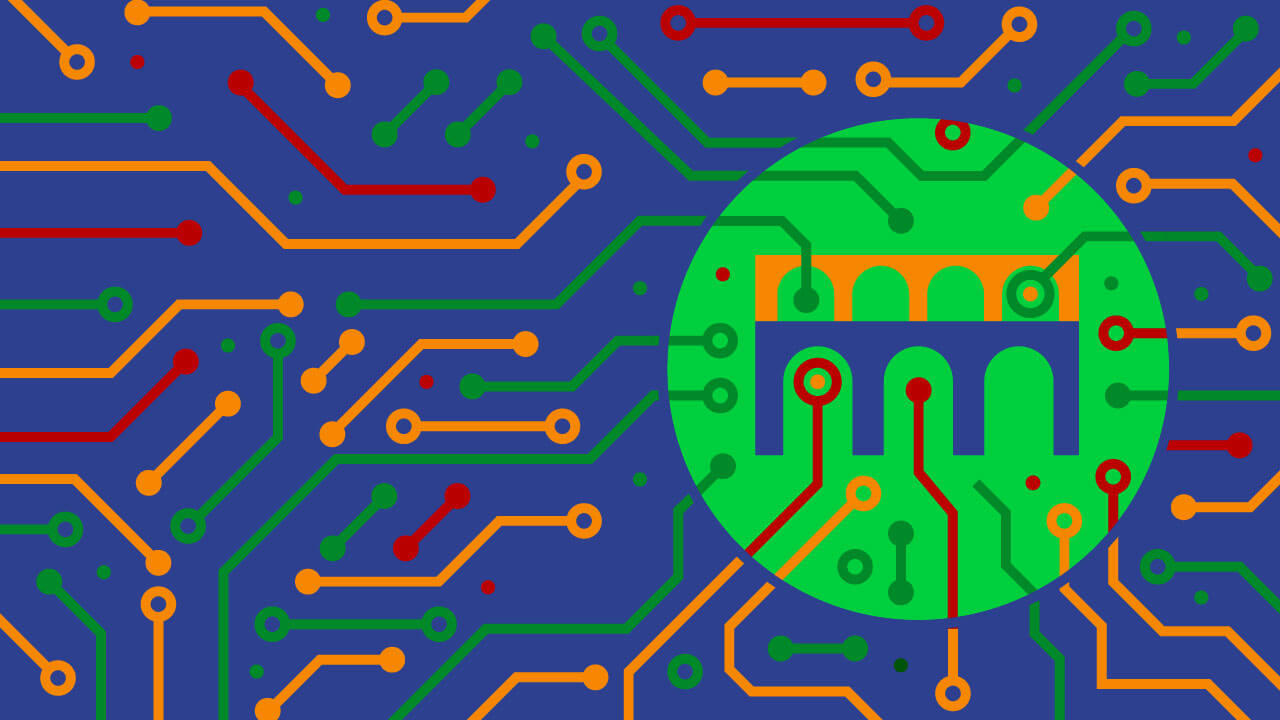
Companies today know that operational insights are inherent in their data. Artificial intelligence (AI) and machine learning (ML) have the power to unlock that value, but data complexity and silos make accessing, analyzing, and acting on stored data difficult. The recently announced IBM ESS 3500 global data platform addresses these challenges, making data delivery for AI workloads faster, and allowing organizations to gain the benefit of their data efficiently.
ESS 3500 brings exciting features that can help organizations overcome serious barriers to unlocking the value of distributed data. Let’s break it down.
It’s All About Speed
“We wanted to have the fastest product on the market, capable of delivering data at sustained speeds greater than our competition so that organizations have access to that data faster than ever before so they can train AI and ML models faster than they ever could before,”
says Scott Baker, CMO, IBM Storage. “We also wanted organizations to have access to data throughout the entirety of their information landscape, including data that resides on non-IBM technology, data that resides in the cloud.”
Businesses are pivoting toward AI and ML to automate processes, and to analyze data and extract information relevant for decision-making. AI and ML models require massive amounts of data to train, and companies need to be able to access that data faster than ever before. ESS 3500 makes that possible.
“Obviously when we’re talking about big data kinds of workloads, AI and ML and even high-performance computing, there’s a continued pivot by organizations to lean in on the GPU kind of data computation,” notes Baker. IBM considered GPU processing in relation to storage, and taking full advantage of the process capabilities with GPU-based computational layers. Giving the computational layer, the GPU, direct access to the underlying storage provides data faster. “The whole reason companies make a financial investment in GPUs is because they want the computational experience to be faster than it ever has been before on a traditional server architecture,” says Baker.
ESS 3500 enables the ability to increase the speed at which AI models transform data so it’s usable by the model by 100% while also cutting down on the number of GPU nodes required by 2x, Baker adds.
Improving Security and Cyberresiliency
Being able to do things faster was one of the primary reasons for ESS 3500, but cyberresiliency is not far behind. “Everything we’re doing within storage thematically will fall into two camps,” says Baker. “Increasing applications performance, and safeguarding and protecting information.”
Baker notes that features built into the technology stack protect data from malicious attacks and keep copies of the data in secure and isolated recovery environments that the organization can extract in order to get the business back up and running in the event of an attack. “We actually extend that capability beyond the primary storage that we’re talking about here into how we’re doing backup,” he adds.
Those resiliency features are based on the NIST framework. “We help organizations understand their attack landscape, the degree of exposure that attack surface has, and what the organization should do to resolve that,” says Baker. This service is vendor agnostic. These tools integrate with IBM security software and allow organizations to detect anomalous behaviors in the information supply chain, and crucially, to deploy an automated response quickly. “Businesses can get back up and running within minutes to hours versus waiting days to weeks using non-IBM solutions,” Baker says.
The Pros and Cons of Unstructured Data
Within the IT industry, experts agree that around 80% of organizational data is unstructured in nature. Such data consumes an enormous amount of capacity, it’s unclassified, may be made up of different file types and often difficult to categorize.
In addition, unstructured data is often strewn throughout the information landscape and may create data silos. The organization must manage extra bits of infrastructure because of it, and ownership and responsibility are often unclear, leaving the questions of data strategies, security and protection unanswered.
“Fragmented ownership in technology silos exacerbates data growth, and so it becomes very circular in nature,” says Baker. “Imagine your body if it never got rid of skin cells when they died. Imagine what you might look like if that were the case.”
Even with all of those negative aspects of unstructured data, it has value. “The nice thing about unstructured data is the fact you’re not bound by the data set itself and how the application in question wrote it. It can be very complicated in nature, but it can also be very rich in terms of what’s inside of it,” Baker explains. Such data, when fed into AI and ML models, can make them much better at understanding human-to-machine interaction that doesn’t usually come from structured data sources.
Unstructured data also provides a measure of creative freedom that isn’t there with semi-structured or structured data sets. “Unstructured data opens the world up to information exchange between humans that you wouldn’t otherwise get with structured data without the access via an application stack,” Baker says. The ease of a conversation can happen in an unstructured form of data, without the need of a back-office application, exporting a data set, and so on.
Storage, Performance and Delivery in the Real World
One real-world example of how the features built into ES 3500 are beneficial can be found in how one customer applied them to the development of self-driving cars.
Self-driving cars require massive numbers of sensors running. This organization uses the information from those sensors to create mesh networks that capture information about the vehicle, the road conditions, obstacles, other vehicles and more. That huge amount of information must be stored somewhere. For self-driving cars, data needs to be scalable and immediately accessible to AI and ML algorithms, regardless of how it was collected or the protocol that was used to generate it.
The information is used to help AI and ML learn things like the difference between a plastic bag and a small child in the car’s path of motion. With the ability to store and access data quickly, various AI models can consume information across whatever kind of protocol is required. Further, the company can train AI models in real time and push information back into the car in a continuous update cycle and across different testing activities.
“Once an AI model is trained,” says Baker, “then it understands the difference between a small child and a plastic bag and it uses this mesh network relationship with other cars to push the updated model. You’re using ESS 3500 to support the model-training and to affect all of the other autonomous vehicles and the tests going on with them. They are actually using this as a deep learning foundation.”
The Future of Storage
Baker has a few predictions about the future of storage. For example, he expects computational intelligence being driven down into the layers of products more than ever before and for consumption of products and services to happen in whatever way customers prefer whether buying and owning, renting, or through an as-a-service model.
The cloud architecture will continue to grow, and storage will continue to become more consistent regardless of architecture with operational capabilities the same regardless of whether the tools are on-premises or in the cloud. Baker also expects to see more multicloud architectures. Companies may be required to store data for certain periods of time, but extracting it may be prohibitively expensive so paying a maintenance fee to remain in compliance could become more common.
An expansion of data privacy laws is another likely scenario. “GDPR set the ball rolling,” says Baker, adding, “I think we’re going to see businesses having more responsibility for the data,” which will lead to storage vendors having to think about security and resiliency and governance and compliance.
Baker’s final prediction is that the push toward containerization will continue. “I think this is now the opportunity for containers to begin to step in and overtake the virtualization wave that we’ve been living in over the last 10-15 years, where more organizations are going to use containers and lean in on their portability for applications.”