Quantum Computing, the Mainframe’s New Horizon
A few of the ways the mainframe can leverage the unique properties of qubits for transactions and large-batch processing
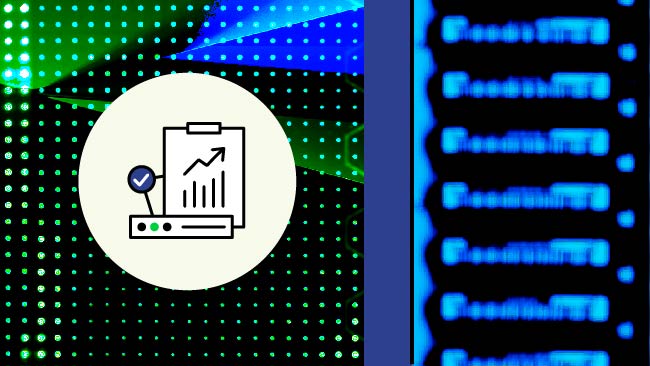
The mainframe has been the beast of enterprise computing for decades but has faced challenges in recent years as cloud computing has gained prominence. The advent of quantum computing, however, brings a new opportunity for the mainframe to strengthen its position as a critical component of modern IT infrastructure.
Quantum computing, a revolutionary technology that harnesses the principles of quantum mechanics, offers the potential to solve complex problems that are impossible for classical mainframe computers. By leveraging the unique properties of qubits, quantum computers can perform certain calculations exponentially faster than their classical counterparts. This computational power has significant implications for various industries, including finance, pharmaceuticals and science in general.
While the mainframe has traditionally been associated with large-scale batch processing and transaction processing, it can also be a valuable platform for exploring and leveraging quantum computing capabilities. Here are some ways quantum computing can benefit the mainframe:
Applications Acceleration
Many mainframe applications are computationally intensive, involving complex algorithms and large data sets. Quantum computing can significantly accelerate the execution of these applications, improving performance and reducing processing times. For example, quantum algorithms could be used to optimize financial modeling, drug discovery simulations or supply chain optimization tasks.
Enhanced Data Analytics
The mainframe often serves as a repository for vast amounts of data. Quantum computing can be used to extract valuable insights from this data more efficiently. Quantum machine learning algorithms can analyze large datasets to identify patterns, trends, and anomalies that would be difficult to detect using traditional methods. This can lead to improved decision-making and predictive analytics.
Quantum-Safe Cryptography
As quantum computers become more powerful, they pose a threat to existing cryptographic systems. Quantum-safe cryptography, which is resistant to attacks from quantum computers, is essential for protecting sensitive data. The mainframe, with its robust security features, can serve as a platform for implementing and managing quantum-safe cryptographic algorithms.
Integrating With Hybrid Cloud Environments
Quantum computing can be integrated with hybrid cloud environments, where workloads are distributed across on-premises and cloud-based infrastructure. The mainframe can act as a central hub for managing quantum computing resources and integrating them with existing cloud-based applications. This hybrid approach can give organizations the flexibility and scalability required to meet their evolving needs.
Driving Innovation in Quantum Computing Research
Mainframes can be valuable tools for researchers working on quantum computing. They can provide a stable and reliable platform for testing and developing quantum algorithms, as well as for simulating quantum systems. By leveraging the mainframe’s computational power and storage capabilities, researchers can accelerate their progress in this emerging field.
The key is tying these two incredible technologies together to help each other and enable each technology to its full potential. Consider the following potential use cases.
Use Case 1: High-Speed Transaction Processing
A global financial institution processes millions of transactions per second, such as stock trades and payments. Mainframes, renowned for their ability to efficiently manage high-volume data streams, excel at handling this transaction load. However, when it comes to more complex tasks like real-time optimization and risk analysis, the sheer scale of operations pushes classical systems to their limits. In this scenario, quantum computing could address these challenges by leveraging its superior optimization algorithms.
Quantum computers are particularly adept at solving complex optimization problems, which are crucial in high-speed transaction systems. One such algorithm, the Quantum Approximate Optimization Algorithm (QAOA), offers significant advantages over classical algorithms by efficiently exploring vast solution spaces. Similarly, quantum annealing can be used to find near-optimal solutions in challenging optimization scenarios. In this use case, QAOA or quantum annealing would help in routing transactions more efficiently, reducing bottlenecks and optimizing resource utilization.
The primary goal here is to enhance transaction routing and risk management, where even minor inefficiencies can have significant financial implications. Quantum algorithms could analyze large volumes of data in real-time to determine optimal transaction paths, manage liquidity risks and mitigate compliance-related vulnerabilities—much faster than classical methods.
Mainframes still play a critical role in data preprocessing. Before data can be processed by quantum systems, mainframes aggregate and preprocess it to identify transaction patterns and extract risk indicators. This step ensures the data is structured in a way that quantum computers can handle effectively.
The key data sets involved include transaction patterns, risk metrics and compliance data. Historical transaction patterns help detect irregularities and predict future trends, while real-time streams allow for immediate responses to market fluctuations. Quantum computing enhances the financial institution’s ability to process this data quickly, minimizing risk and maximizing profit potential.
Mainframes classify and preprocess transaction data based on operational metrics, such as risk thresholds, compliance scores and transaction volume trends. This information is then summarized and transformed into quantum-compatible formats, such as feature vectors representing different transaction scenarios. The preprocessing reduces the complexity of the data while preserving key indicators for further quantum analysis.
Quantum systems then process the data, performing advanced optimization and risk evaluation. However, quantum processors have limitations in terms of data storage, meaning only the most critical features or variables are selected for quantum processing.
Use Case 2: Large-Batch Processing
In industries like retail, large-batch processing is critical for managing daily operations, from sales analytics to supply chain logistics. Mainframes currently handle these massive data loads efficiently but often face difficulties optimizing supply chain management or providing actionable insights in predictive analytics, especially when dealing with enormous volumes of data. Quantum computing can overcome these challenges by applying advanced machine learning algorithms to optimize these processes.
Quantum algorithms, such as Quantum Support Vector Machines (QSVM) and the Variational Quantum Eigensolver (VQE), are highly effective at pattern recognition and predictive analytics. QSVM, for instance, can classify complex data patterns more efficiently than classical support vector machines, making it well suited for predicting customer behavior or sales trends. VQE can be used for optimization tasks in supply chain management by calculating the most efficient logistics routes and resource allocation strategies.
Quantum machine learning algorithms could provide more accurate demand forecasts, optimize inventory levels and improve the efficiency of supply chains by analyzing complex correlations in large datasets. The ability to process such intricate data relationships offers a significant improvement over classical systems, particularly in retail sectors where supply and demand fluctuate rapidly.
As with the high-speed transaction case, data preprocessing is handled by mainframes before being passed on to quantum processors. The mainframes aggregate and clean large volumes of sales data, inventory records and supply chain logistics. This preprocessing step includes tasks like data normalization, which ensures that the data is ready for quantum analysis.
The critical datasets include sales records, inventory levels and logistics information. Quantum computers could analyze this data to provide insights into future inventory needs, helping retailers better manage stock and reduce the risk of overstocking or understocking. The relevant data subsets are transformed into quantum-friendly formats, with dimensionality reduction applied where necessary to fit the constraints of the quantum system. Classical systems may further refine the data to create feature vectors or other structured formats. These are fed into quantum algorithms, enabling the quantum processor to perform tasks such as optimizing resource allocation in the supply chain.
Defining the Future
Quantum computing’s potential lies in its ability to tackle complex optimization and predictive analytics challenges that are difficult for classical systems. By working in tandem with mainframes, quantum computing can complement existing infrastructure, providing new computational capabilities that significantly enhance performance. The future of quantum-mainframe integration promises to reshape industries that rely on processing vast volumes of data at rapid speeds, increasing business agility and contributing to more successful business outcomes. As quantum computing technology continues to advance, the mainframe’s role as a critical component of enterprise infrastructure is poised for a resurgence, driven by the transformative potential of this groundbreaking technology.