IBM Storage Ceph: Using Software-Defined Storage to Harness the Power of AI
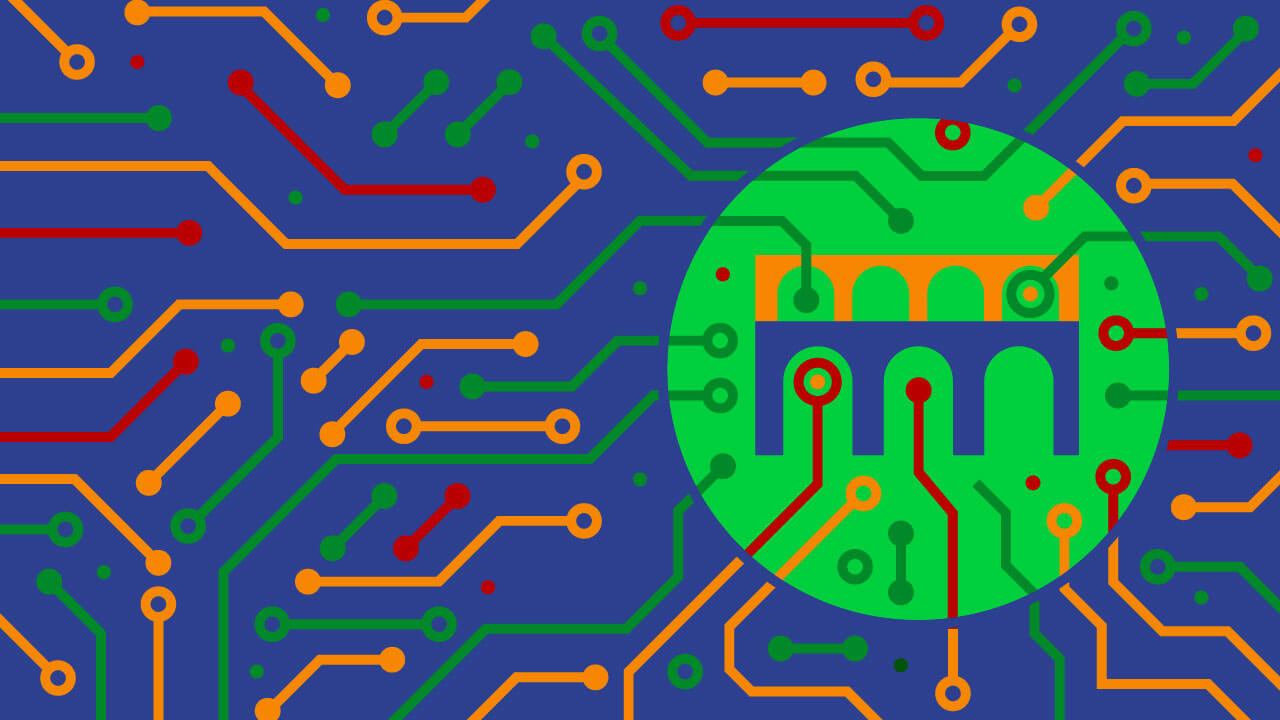
In the future, the year 2023 is likely to be remembered as the year generative artificial intelligence (AI) changed business. Enterprises are finding new ways to derive value from AI, and doing so is increasingly imperative. One of the most important aspects of AI, though, is making sure that the data it’s using is relevant to the enterprise using it. In other words, simply using an off-the-shelf foundational model like ChatGPT won’t yield the most useful results. Instead, each organization must apply their own data to the model. Although that sounds simple enough, the enormous amount of unstructured data in various formats and stored in different ways makes actually using data more complicated.
IBM Storage Ceph 7.0
On December 8, 2023, IBM released the first major update to IBM Storage Ceph, its object, block and file storage system. The update provides improved performance for object storage for machine learning and data analytics for three defined S3 Select formats, CSV, JSON and Parquet.
It also provides bucket granularity, which is useful for selective replication in situations where edge, colocations or multiple branch offices are involved. Additionally, the update gives clients the ability to move data based on specific policy thresholds.
Sam Werner, vice president of product management, IBM Storage, discussed IBM Storage Ceph 7.0 with TechChannel, and elaborated on why software-defined storage is growing in the age of AI.
Jumbled, Unstructured Data
For years, organizations have been collecting data and storing it in data warehouses. Structured data can be used for queries of multiple databases, but structured data makes up only a small percentage of data in storage. Some 80%–90% of the world’s data is unstructured. The exponential growth of data continues, as well, and most of it will continue to be unstructured.
Using AI allows for the massive amount of unstructured data files in all different formats to provide value. “Now you have the opportunity to really learn something new that you couldn’t before with traditional analytics,” says Werner. “But to do that, you need a way to organize all of the data.”
The challenge for companies lies in organizing unstructured data in a way that provides real business value.
Right now, organizations have data sitting in public clouds, data centers and on the edge. It’s everywhere, and it’s generally not well organized. Moving data is challenging on multiple levels from physics to cost. Accessing data where it is and where it’s being created is the best solution.
Software vendors are working on solutions, but it comes down to organization. How can unstructured data be quickly organized and managed so that its value is realized?
If you imagine looking through an enormous pile of clean laundry in a jumble, trying to find one sock, you know how important some sort of organization is. For AI to provide useful insights, being able to find the right information is crucial.
Paul Kirvan, writing for TechTarget, says object storage is the right solution. “Metadata containing attributes of the contents of the object helps AI/ML systems find the data needed. An identifier helps find the correct object,” he writes.
Another point to consider is the continual and exponential growth of data, alongside the expanding use of AI. Organizations need to accelerate how they ingest data, cleanse it and classify it. “In order to do that,” says Werner, “they will probably need some kind of software defined approach that gives them the flexibility and the elasticity of the infrastructure to support the data in multiple locations.”
The Infrastructure Challenge
One of the biggest challenges to adopting a more flexible, elastic storage method is one of infrastructure. Currently, most enterprises have vast amounts of unstructured data sitting on legacy appliances. “They use a NAS protocol,” says Werner, “and nobody builds applications anymore for a NAS protocol.”
Object storage, by design, scales out without the contention that occurs on a traditional file-like platform because the metadata is maintained with the objects. Along with that scalability, object storage uses a RESTful API, making it easy to connect to the data from many different applications. This is what people use in public clouds, whether it’s AWS, Azure or IBM Cloud. “You put your data—all of your unstructured data—to an object storage and you run your application on block storage,” says Werner.
Enterprises want the same model on-prem. It’s often less costly, and there’s quite a lot of data that must stay on-prem due to security or data sovereignty rules or regulation, or some other concern. The problem is that infrastructure for on-prem is different in that people ask for the absolute gold standard—lowest latency, highest performance, highest availability. All of that makes the infrastructure rigid and difficult to scale up or down and is totally optimized to a specific workload.
The difference with the cloud is that everyone tends to accept that it’s just going to be generic block storage. Application developers quickly become frustrated with the on-prem experience because of the lack of flexibility. The way that applications are built for on-prem and for cloud are different architectures, as well, creating another issue.
Werner believes that the solution is a common architecture. “It doesn’t have to be the exact same infrastructure, but it has to be the same architecture,” he says, adding that’s the goal of the most recent iteration of Ceph. “Ceph is a scale out software-defined storage platform that offers block, file and object all on one storage platform.”
Limiting the Risk
Copying and moving data is expensive and creates unnecessary risk. At the core of limiting risk is data management. Metadata management along with a clear understanding of where all the data is and a software defined approach to support data in multiple locations help.
“But really, ideally,” says Werner, “what they want to do is get a common platform so the way they manage data in the cloud and at the edge is the same—that way, they don’t have different operating models everywhere they are. You want to bring AI to where the data is, and you want a consistent approach in how you’re managing it.”
Leaving data where it is and allowing AI to access it only as necessary reduces risk. In a world of increasing cyber risk, any way to reduce exposure while still getting value makes good business sense.
From Consideration to Application
When it comes to software-defined storage, complexity has always been a challenge. It’s the kind of thing that people have talked about for years, but the questions of deployment and management represented nearly insurmountable barriers. Things are changing now, and vendors are reaching a point where this type of storage is more consumable.
As of now enterprises are shifting, while most of the market continues to use traditional arrays, but that is beginning to change, too. According to Mordor Intelligence, the software-defined storage market is expected to grow by 25.8% CAGR through 2027. “This year, I think we’re going to see significant disruption,” notes Werner.
For an enterprise to move successfully to a software-defined storage approach, a cultural shift within the organization must happen. Werner says more progressive companies that are changing how they do IT operations to embrace platform engineering are closer to fully switching to software-defined storage.
“Ultimately, all enterprises that want to be successful in this transformation and stay competitive are going to have to move to a different model of managing infrastructure and move to this platform approach,” says Werner.