Why Today’s AI Is Failing
iTech-Ed Ltd’s Trevor Eddolls on artificial generalized intelligence and what AI experts can learn from analyzing the human brain
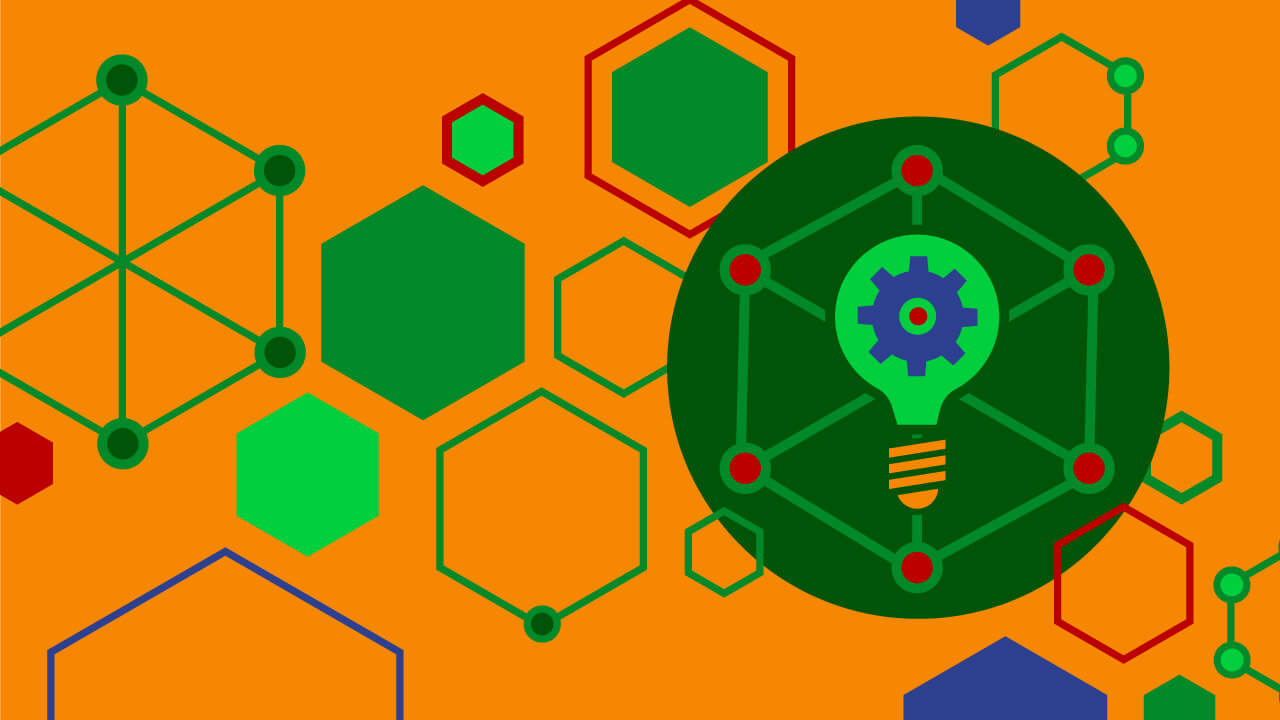
You might think that’s a stupid title for an article when AI is clearly everywhere. So many people have at least had a go with ChatGPT or Gemini (Bard, as was). There are hundreds of articles and news reports about AI. Governments are looking for ways to restrict the use of AI. Why would anyone suggest that it’s not successful?
The History of AI
Before I answer that question, let me turn time back to the late 1970s. For people who weren’t around in those days, it was a time when there were mainframe computers, and it was the start of specialized computing. Organizations were beginning to buy word processing computers and other devices that had a single function and weren’t connected to any other device. It wasn’t until a few years later that companies began buying PCs that could handle all kinds of applications—things like word processing, spreadsheets and drawing apps—on one device. The 1970s marked the move away from single-function computers to more generalized ones that launched the world of computing that we know today, including phones and tablets.
So, let’s look at the world of AI at that time. Many may remember IBM’s Deep Blue computer beating world chess champion, Gary Kasparov, over six games in 1997. People may have heard of IBM’s success at the TV game called Jeopardy. Today’s AI is very similar to the computing environment of the 1970s, with highly specialized machines that were great at what they did but unable to do anything else.
Training Modern AI
AI has advanced significantly since then. Today, generative AI can produce or generate high-quality content, including text, images or videos. However, a limiting factor remains: the fact that the AI must be pretrained and, although it is very good at what it has been trained on, it is useless at anything else.
Training AI involves feeding it data and letting it try to recognize patterns, then make decisions or predictions. This can be done with:
- Reinforcement learning: The computer application gets rewarded when it gets something right. It’s used in gaming, robotics and autonomous vehicles.
- Unsupervised learning: The computer explores the data, finding patterns and relationships of its own.
- Supervised learning: The software is given labeled data, checking its answers against the ones given to modify its algorithms appropriately.
But, again, the AI only knows what it’s been trained on, and it can’t learn anything else. That’s the big difference between artificial intelligence and human intelligence.
AI and the Brain
Consider how a young child learns so much in their first year by exploring and trying things on their own. As they get older, they can be taught, but they will still go off on their own and find things out. Compare that to AI, which can’t initiate its own learning. It lacks interest or motivation. What’s missing is working artificial generalized intelligence (AGI).
To better understand the difference between AI and the brain, let’s consider how the human brain works. It is essentially divided into two parts. The first part is very quick at reacting and is focused on activities such as feeding, fight or flight and reproductive behavior. The second part is the rational, or logical, part that seems to be made up of small, identical components called neurons, which are grouped into cortical columns. There are around 150,000 cortical columns in the human brain. The ability to grow certain parts of the brain by adding more of these tiny components has allowed humans to evolve to their current form.
The rational brain makes multiple simultaneous predictions about what it is about to see, hear and feel. To be able to make predictions, the brain must learn what’s normal in its environment using past experiences. It creates a model of the world as the person moves and notices how sensory inputs change. With each movement, the rational brain can predict what the next sensation will be. If the prediction isn’t correct, the model in the brain is updated.
For an AI model, that means creating a way that the AI can learn by itself, create a model of the world that it lives in and, in order to work even faster, predict what is likely to happen next in any situation. Also, if a prediction is wrong, the AI can update its model of the world. And that whole ability moves us from a narrow AI to a generalized AGI.
Exploring AI Through a Neuroscience Lens
The Thousand Brains Theory of Intelligence assumes cortical columns are not only learning machines, but they are predictive. It also assumes that they do this using reference frames, which model everything a person knows and are found everywhere in the rational brain.
If people working with AI could take this model of the brain and use it to create a new type of AI machine, they would have solved the problem of how to create AGI. Because of the predictive nature of its equivalent to cortical columns, AGI could quickly give answers and learn new things itself without needing training. It could also become more intelligent by adding more cortical columns.
Currently, there is narrow or weak AI that is brilliant at one task (like being a virtual assistant) or a range of similar tasks, which is like computing was back in the late 1970s. And there’s general or strong AI, which aims to replicate human intelligence. Currently, companies like Anthropic, DeepMind, OpenAI and many others are working on creating an AGI.
What I’m suggesting (and finally answering my own question) is that this aim won’t be successfully achieved until something similar to the cortical columns found in the brain can be implemented in hardware or software. If that happens, we’ll have fully working AGI that would be able to seek out its own information, which it could use to make rational decisions or anticipate and help prevent potentially disastrous situations. A single AGI could do far more than any more focused narrow AI can now, and that could be a great benefit to the general population. I expect it will be as commonplace as laptops and phones are today.
You can find out more about these ideas in Jeff Hawkins’ book, A Thousand Brains: A New Theory of Intelligence.