Data Cleansing is Critical to Business Success
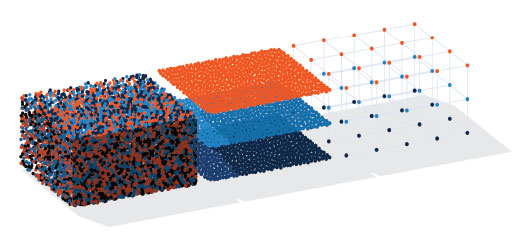
Regardless of their size, businesses are increasingly reliant on data analytics to maximize profit and efficiency.
But with so much data now available through all sorts of technical applications, how does a business know which numbers to trust? And what happens if a number is off by a decimal point, or a piece of information gets filed under the wrong column of a spreadsheet? The results of such an error could be catastrophic.
That’s why data cleansing is becoming an increasingly useful tool for organizations that use information to conduct their operations. Simply put, data cleansing is a way for a business to make certain its information is as accurate as possible and easily accessible for its employees and customers.
Data cleansing doesn’t just involve eliminating data that might be inaccurate or out of date; it also means organizing the data in an efficient manner and reducing the possibility of other errors, such as duplicate, missing and misfiled data.
IBM, through offerings such as IBM Watson* Information Suite and IBM InfoSphere* QualityStage, is among the industry leaders when it comes to data cleansing.
Linton Ward, Distinguished Engineer, OpenPower solutions, notes that data must be accurate before model-building has even begun, because bad data can ruin the foundation of the model.
“The reason you want to do data cleansing and manipulation of the data prior to model building is that if you build a predictive model on bad data, then your model is potentially bad as well,” he says. “I hate to use the phrase ‘garbage in, garbage out,’ but it’s very akin to that thought. Data preparation is absolutely essential to good answers and results that you can count on.”
“Data is one of a company’s key assets, and being able to leverage that is really important. Being able to look at how they can get a coherent and trustable set of that data infused into their analytic functions is really key.”
–Mythili Venkatakrishnan, Distinguished Engineer, IBM
Data Fusion
Ward says many data sets come from multiple sources, so it’s important that the data is clean once those sources are fused. That means eliminating duplications or finding missing data.
“When you begin to augment tabular data or numerical data with text data or data from other sources, I now begin to build more context around those quantitative answers and so now I can begin to add more power to the predictive nature,” Ward says.
Ward also notes that adding non-numerical information, such as social media comments, can give the user more context to augment the raw numbers. For example, a business can analyze social media feeds to assess which items might be more popular among consumers—something that may not be as apparent as using raw numbers.
A Key Business Asset
Because of the relative novelty of data cleansing, organizations often don’t understand its value in getting a more complete picture of their business.
Mythili Venkatakrishnan, Distinguished Engineer, IBM, has worked with several companies that needed a better understanding of the value of data cleansing. “We’ve worked with a lot of clients that, in terms of enabling analytics with their core transactional data, didn’t realize that they could actually use this data for that reason—everything from improving decision-making on claims processing to being able to detect fraud situations, etc.,” she says.
A company’s strength lies in its data, though not every company realizes this. “Data is one of a company’s key assets, and being able to leverage that is really important. Being able to look at how they can get a coherent and trustable set of that data infused into their analytic functions is really key,” Venkatakrishnan says. “If they don’t, they are going to make bad business decisions. They’re going to possibly open themselves up to ethical issues, legal issues, or compliance and regulatory issues.”
Using AI
With some entities operating in real-time situations, the opportunity for human/computer checks and balances may not exist. Artificial intelligence (AI) can be a key factor in bridging the gap to provide more accurate data sets without human supervision.
Many of today’s new technologies—from self-driving vehicles to real-time stock trading—use closed-loop systems, and humans using the technology need to be able to trust the data that’s driving them. “I need to be able to count on this model, which means I need to be able to discover that this data was good and I need to be able to use modern data science models,” Ward says.
For example, fair-lending policies in housing markets are largely dictated by available data. If a data set is skewed in some way in favor of or against a certain demographic group, it could lead to unfair lending policies in a community.
“To say to a regulator, ‘We’re being fair here,’ you have to be able to back that up and show how we develop it,” she says. “If you’re going to use AI and cognitive as part of your decision, here’s the data. Here is how it was appropriately distributed across all of the constituencies we serve and here’s how you know how we developed the model.”
ETL Software and Data Cleansing
Traditionally, engineers have used Extract, Transform and Loading (ETL) software for data cleansing. However, new categories of tools use open-source software to increase the effectiveness of data cleansing.
IBM toolsets use ETL components for cleaning data, and use open-source materials that can track the source of data and capture metadata. Watson, for example, uses a technique called tooling emerging, which assists in automated discovery.
“Automatic tooling for data discovery, analysis and comparison of data to pop up where I need it and identify where I need more attention is a big piece of this,” Ward says.
Several IBM InfoSphere products can also help with data sorting, including Information Server for Quality Data, QualityStage*, BigQuality and Information Analyzer. In addition, IBM Watson Discover makes it easy to build cognitive, cloud-based exploration applications that unlock actionable insights hidden in unstructured data.
“Data preparation is absolutely essential to good answers and results that you can count on.”
—Linton Ward, Distinguished Engineer, OpenPower solutions
A Supply Chain of Insight
Ultimately, it’s important for agencies and businesses to learn the value of their data and how to keep it accurate. “People are shocked when they find out what they can actually do with the data they already have,” Ward says. “The gap between potential and reality is still pretty large for most clients. I use the term ‘data productivity,’ which is a measure of how much of the data is in productive use for actionable insights.”
General estimates of data productivity in IT are very low, below 10 percent. But data cleansing can make all the difference. “There’s a shift occurring for these kinds of use cases. Rather than becoming a cost center, IT is becoming a strategic weapon for differentiation,” Ward says. “Data cleansing is an unavoidable first step in the supply chain of insight.”